Heart disease remains a leading cause of mortality worldwide, emphasizing the critical need for innovative approaches to prediction and prevention. In recent years, machine learning has emerged as a powerful tool in healthcare, offering unprecedented insights and capabilities in disease prediction. In this article, we delve into the groundbreaking advancements in heart disease prediction using machine learning algorithms, highlighting their potential to revolutionize cardiovascular healthcare.
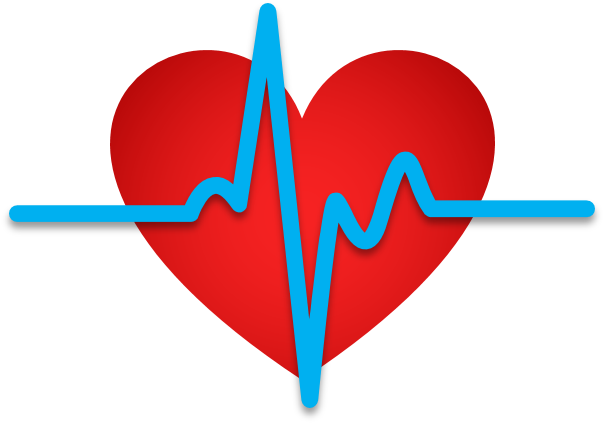
Revolutionizing Heart Disease Prediction with Machine Learning
Predicting heart disease accurately is a multifaceted challenge that involves analyzing numerous risk factors, including genetic predispositions, lifestyle choices, medical history, and physiological indicators. Traditional methods often rely on manual assessment by healthcare professionals, which may be subjective and prone to human error. Additionally, these approaches may not fully leverage the wealth of data available in electronic health records (EHRs) and medical imaging.
The Role of Machine Learning
Machine learning offers a paradigm shift in heart disease prediction by harnessing the power of data analytics and computational algorithms. By training models on vast datasets encompassing diverse patient profiles and health metrics, machine learning algorithms can identify intricate patterns and correlations that may elude human observation. This enables more accurate risk stratification and personalized interventions tailored to individual patients.
Types of Machine Learning Algorithms
1. Supervised Learning
Supervised learning algorithms, such as support vector machines (SVM) and random forests, learn from labeled data to make predictions. In the context of heart disease prediction, these algorithms analyze historical patient data, including demographics, medical history, laboratory tests, and imaging studies, to predict the likelihood of developing cardiovascular complications.
2. Unsupervised Learning
Unsupervised learning algorithms, such as clustering and dimensionality reduction, extract meaningful insights from unlabeled data. In heart disease prediction, these algorithms can uncover hidden patterns within large datasets, facilitating risk stratification and early detection of high-risk individuals based on shared characteristics or risk profiles.
3. Deep Learning
Deep learning algorithms, particularly convolutional neural networks (CNNs) and recurrent neural networks (RNNs), excel in processing complex, high-dimensional data such as medical images, electrocardiograms (ECGs), and genomic sequences. By automatically learning hierarchical representations of data, deep learning models can discern subtle abnormalities and biomarkers indicative of underlying cardiovascular pathology.
Advantages of Machine Learning in Heart Disease Prediction
1. Enhanced Accuracy
Machine learning models can integrate a multitude of variables and data sources to generate more precise predictions of heart disease risk. By leveraging advanced algorithms and ensemble techniques, these models can achieve superior accuracy compared to traditional risk assessment tools.
2. Personalized Risk Assessment
One of the key strengths of machine learning is its ability to provide personalized risk assessment based on individual patient characteristics and health profiles. By considering unique combinations of risk factors and biomarkers, machine learning algorithms can tailor preventive strategies and interventions to each patient’s specific needs.
3. Early Detection and Intervention
Machine learning algorithms excel in early detection of cardiovascular abnormalities and preclinical manifestations of heart disease. By analyzing subtle deviations from normal physiological patterns, these algorithms can identify individuals at heightened risk of developing heart disease long before symptoms manifest, enabling timely intervention and lifestyle modifications.
Challenges and Considerations
While machine learning holds immense promise in revolutionizing heart disease prediction, several challenges and considerations must be addressed:
1. Data Quality and Bias
The quality and representativeness of training data are paramount to the performance and generalizability of machine learning models. Biases inherent in healthcare data, such as disparities in access to care and underrepresentation of certain demographics, can adversely affect model accuracy and exacerbate health inequalities.
2. Interpretability and Transparency
The inherent complexity of machine learning models, particularly deep learning architectures, can hinder their interpretability and transparency. Clinicians and patients may be skeptical of black-box algorithms that lack explainability, emphasizing the importance of developing interpretable models that provide insights into decision-making processes.
3. Regulatory and Ethical Considerations
The integration of machine learning algorithms into clinical practice raises ethical, legal, and regulatory challenges regarding data privacy, informed consent, and algorithmic accountability. Regulatory frameworks must evolve to ensure the responsible development, validation, and deployment of machine learning-based predictive models in healthcare settings.
Future Directions
Despite these challenges, the future of heart disease prediction using machine learning is incredibly promising. Ongoing research efforts aim to address current limitations and enhance the robustness, interpretability, and ethical implications of machine learning models in cardiovascular healthcare. Collaborative initiatives between clinicians, data scientists, policymakers, and patients are essential to realizing the full potential of machine learning in improving cardiovascular outcomes and reducing the global burden of heart disease.
In conclusion, machine learning represents a transformative approach to heart disease prediction, offering unprecedented accuracy, personalization, and early detection capabilities. By harnessing the power of data analytics and computational algorithms, healthcare providers can usher in a new era of precision medicine that revolutionizes cardiovascular care and saves lives.